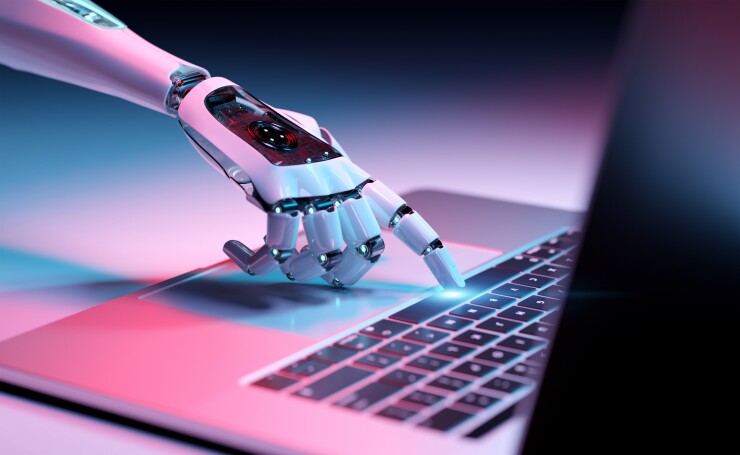
In the rapidly evolving landscape of artificial intelligence for banking, the past 18 months have produced a fascinating evolution in the technology, the players and overall industry perception.
Even with its ambitious vision to transform the banking industry and its noteworthy early successes, generative AI has one well-known drawback: implicit bias, which poses a risk if unaddressed. For example, on Feb. 26, Google's parent company, Alphabet, saw its market capitalization drop by the equivalent of Disney's total net worth after its Gemini product was widely criticized for its issues with bias.
Is AI bias worth addressing? Is it worth addressing in banking? Absolutely. But what exactly is the problem and how does it get fixed?
Let's begin by discussing the expectations of relevancy and freshness of the training data, particularly in the context of written content. By its very nature, once a word has been laid down to paper (or to electronic format) it is already an expression of the past.
Even if it was only written a week ago, it is now weekold news. This fundamental principle of relevancy and freshness in human communication particularly affects large language models, the brains behind generative AI. The training data that LLMs require combines large amounts of internet text from various time periods.
This text reflects different societal positions on various topics and is written in the language of those times. We can then say the LLM exhibits "bias" as a way of simplifying the problem. All cultures have explicit and implicit cultural biases. We notice the text is inappropriate because its bias is out of touch with our current societal perceptions, meaning LLMs are by definition being trained on outdated information.
Sometimes the bias can be easy to identify and easily fixed. For example, the large training text might include toxic or hateful dialogue, in which case that text is identified and removed.
For wide adoption of LLMs in banking, removing these biases is not only needed but also legally required. Producing customer communications with a gender or racial bias will clearly find pushback from customers and regulators. Most of the training data employed in LLMs is from the 1990s and 2000s when the culture of sharing text freely on the Internet was commonplace. Nowadays, more content is in images and video or behind paywalls.
Fast forward to 2024, our current society has significantly changed its views in many of these areas. Thus, at the very least, a tight human and regulatory oversight for these types of sensitivities is recommended.
Furthermore, cultural bias can be difficult to perceive for individuals immersed in a given culture. It is part of the "operating system" of the society. There are a number of recent technical advances that enable adjusting the LLM bias to conform to current times. It all starts by identifying the existing biases in the system and then using humans to indicate which variations in a text are to be preferred. This is the method used by OpenAI's ChatGPT as well as other leading LLMs to add guardrails to overcome some of the existing bias. This process is very expensive in terms of both personnel and computer time.
In the world of banking, this process needs to be enhanced to prevent LLMs from being used for blatantly illegal activities, such as impersonation, to obtain a loan. Implementing guardrails is an approximation, and the process should be carefully managed as it is prone to overcorrection. This issue contributing to Alphabet's value loss mentioned above was about their new product, Gemini, overcorrecting to the point of generating historically inaccurate iconography of the U.S. Founding Fathers.
Addressing implicit bias must start at the source. There is a growing understanding in the world of generative AI that the companies that train and build their LLMs on high-quality human-curated data and text, rather than large amounts of random data and text, will provide the most value to their customers.
In financial services, it is imperative to partner with vendors that use high-quality, banking-specific data sources to help mitigate the risk of implicit bias in the AI systems being developed.
Addressing biases necessitates a shift toward custom LLMs that are tailored for industry specific needs. An LLM that is built for banking offers the same experiences and features as the larger, general purpose LLM while also meeting the banking industry's requirements for accuracy, transparency, trust and customization.
These models are not only more cost-effective to create and operate, but they also provide better performance compared to general-purpose LLMs. Moreover, as generative AI has evolved toward multimodal capabilities, integrating text, image and other data modalities, banks will be able to leverage this capability to analyze diverse types of information and deliver more comprehensive insights.