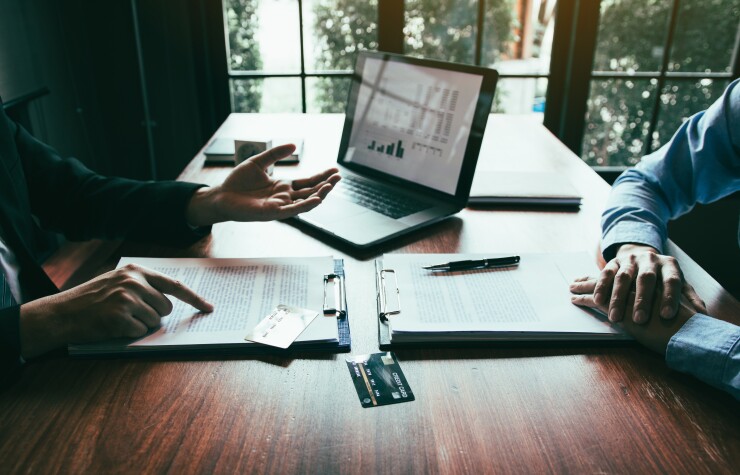
In 2023, generative artificial intelligence took people by storm, and they used it to write stories, make images and find information more easily. Business, particularly in regulated industries like financial services, generally proceeded more slowly, with proof-of-concepts, pilots and testing to determine the right use-cases to prioritize and scale.
The financial services industry is one of the most data-driven industries in the world. Generative AI can be used to analyze this data and generate insights that can help financial institutions make better decisions. It can help banks improve customer experience, increase their efficiency and employee productivity, reduce their costs and develop new and more personalized products and services.
In 2024, I predict that we'll see more banks take their generative AI use-cases from pilots to production for both employees and customers. This will happen in four key ways.
First, the rapid development of generative AI has piqued the interest of business leaders, who are increasingly recognizing its potential to transform their operations and gain a competitive edge. A recent Google Cloud survey found that 96% of banking executives are seeing their C-suite and boards of directors getting more involved in technology and IT decisions due to heightened interest in generative AI.
Generative AI can represent a massive productivity and operational efficiency opportunity. More than a third of executives at national banks (38%) said that generative AI will deliver 61%-to-80% in cost savings over the next five years. That's why business leaders want to ensure that their companies are investing in the right technologies to take advantage of generative AI — whether it's for creating personalized customer experiences and automating tasks or developing new products and services.
Next, we are seeing an explosive growth in generative AI models. Generative AI trains on large amounts of data. These large language models, or LLMs, are the driving force behind generative AI. There have been an increasing number of them recently, differentiated by what they train on, how much they cost to operate and what kind of specialized information they cover.
With much of the financial services industry having its own vocabulary and context, we'll see an increase in fine-tuned LLMs which are pretrained language models that have been further trained on a smaller, more specific dataset of text and code. This allows for the model to better understand and respond to prompts and queries related to a particular topic or domain like regulatory changes or financial reporting standards.
Bank customers' complaints of sudden account closures track a rise in automated anti-money-laundering decisions and possibly outdated AML rules.
Further, the quality of generative AI outputs is improved by "grounding" the model, which connects generated text to real-world information and context. This means that for every judgment or assessment produced, models can footnote or directly link back to a piece of supporting data. Explainable generative AI models enable financial institutions to transparently explain their decision-making processes to customers, building trust and confidence in the AI-driven services they offer.
In addition to the boom of generative AI models, the push for generative AI adoption is creating new talent demands in the banking sector. Almost half (45%) of bank executives we surveyed said their top challenge for adopting generative AI is the talent and skills gap they face, and at the same time nearly every respondent (99%) said that they are hiring talent to support generative AI initiatives.
As certain rote work becomes more automated, we'll see more upskilling in the workforce and an emergence of new roles that will be in demand. For example, AI quality assurance testers, prompt engineers, AI strategy consultants and AI product managers are some of the top roles that bank executives plan to hire, according to our research. In terms of skill sets, having an understanding of cloud technologies, programming language skills and the intersection of business and AI will be increasingly attractive.
Finally, keeping humans in the loop is important for AI in banking because it allows humans to oversee and control the AI systems that are used to make decisions about customers' finances. By keeping humans in the loop, banks can help ensure that AI models are compliant with regulations, mitigate risk and maintain customer trust.
I'd go so far as to say that you'll be able to judge the success of generative AI in 2024 by the number of people throughout the company who are engaging with it.
Who will come out ahead? Clearly, the financial institutions that have spent the last several years modernizing their data foundations are in the best position to leverage their data. Managers who are willing to adjust workflows to take advantage of the productivity gains in customer experience and service. Leaders who see that generative AI can help them understand the company in ways they couldn't before.